1. INTRODUCTION
Climate change and greenhouse gas emissions are among our most significant environmental challenges. Carbon neutrality has emerged as one of the crucial strategies for addressing these challenges. Carbon neutrality involves eliminating net carbon emissions by absorbing or compensating for greenhouse gases in an amount equal to or less than what is produced and emitted (Austin et al., 2020; Larsary et al., 2023).
Forest expansion is a valuable way to achieve carbon neutrality. As trees grow, they absorb carbon dioxide from the atmosphere and capture and store the carbon in their internal fibrous structures (Jang, 2022b; Segaran et al., 2023). Wood also requires considerably less energy to produce and process than concrete or steel. Thus, the sustainable use of wood can significantly assist in achieving the goal of carbon neutrality (Ahn et al., 2021; Ghani and Lee, 2021; Hadi et al., 2022; Han and Lee, 2021b; Jang and Kang, 2022b).
Consequently, the importance of wood science is increasing. Various aspects of wood science have traditionally been studied, including wood physics, wood chemistry, and wood biology (Mai et al., 2022). Recently, with the aim of establishing a sustainable wood industry, the research scope of wood science has been gradually increased, with a focus on aspects such as biomass, composites, sound, and sensitivity engineering (Jang and Kang, 2021; Jekayinfa et al., 2020; Shen et al., 2021; Tian et al., 2021).
More wood science research will be required in the future (Teischinger, 2010). Accordingly, wood science researchers need to interact with researchers from diverse fields to find innovative approaches to building an ecofriendly and sustainable wood industry. As a basis for this work, research trends in wood science need to be examined.
Text mining is a process that combines natural language processing and data mining techniques to extract valuable insights from textual data (Gupta and Lehal, 2009; Rajman and Besançon, 1998). It offers a means to analyze and interpret extensive text data by converting it into organized and meaningful information (Dang and Ahmad, 2014; Liddy, 2000). Text mining enables researchers to uncover patterns, trends, and relationships within scientific literature by employing various computational methods (Sulova et al., 2017).
Text mining techniques have proved useful in understanding research trends in various fields such as medicine, ecology, materials science, and environmental science (Cheng et al., 2022; Farrell et al., 2022; Rabiei et al., 2017; Sakuma et al., 2021; Sumardi et al., 2022; Weston et al., 2019). In Korea, several surveys on timber culture have been analyzed through text mining (Han and Lee, 2021a; Han et al., 2022). Lee and Lee (2022) analyzed domestic and international research trends on pine trees using text mining. The results showed that domestic research of pine trees primarily focused on pine wilt nematodes and various ecological aspects. Overall, though, text mining techniques have rarely been applied to wood science.
The present study was conducted to investigate wood research trends using text mining techniques. Specifically, text mining was applied to articles published in the Journal of the Korean Wood Science and Technology (JKWST; ISSN: 2233-7180) published by the Korean Society of Wood Science and Technology and Journal of Wood Science (JWS; ISSN: 1611-4663) published by the Japan Wood Research Society.
2. MATERIALS and METHODS
This study used abstracts appearing in JKWST and JWS from 2012 to 2022. The 785 articles published in JKWST featured 699 researchers as authors, and the 812 articles published in JWS featured 506 researchers as authors. Therefore, for the purpose of this study, these two journals were considered sufficient and representative of research in their respective countries. Abstracts from both journals were extracted from the SCOPUS database (http://www.scoups.com). Text mining was performed using the open-source KH Coder software (https://sourceforge.net/projects/khc/) developed at Ritsumeikan University in Japan. This software is a verified and trustworthy tool that has been used for text mining analysis in many studies (Jurkus et al., 2022; Sasaki and Ishiwatari, 2022; Sato and Nishizawa, 2019). HD Coder splits sentences into words from the abstract text file (cvs file format) provided by SCOPUS and organizes the results into a database.
The first step in text mining analysis is to preprocess the gathered textual data. This phase usually involves conducting a morphological analysis to classify sentences into various parts of speech. The next step is to extract keywords that highlight important themes and words that appear together in the same paragraph or sentence. Next, various text mining techniques, including keyword network analysis, association analysis, and topic modeling, are employed to understand word characteristics within the document and examine their frequency of occurrence. All of these techniques play a crucial role in uncovering insights and patterns hidden within the text data, and they aid researchers and analysts in better understanding the information contained in the documents (Jung and Lee, 2020).
In this study, the abstracts of the papers were split into words using the “Run preprocessing” command. HD Coder separated the abstract sentences into morpheme units that are tagged by part-of-speech units. Next, “Stopwords” and “Compound words” were processed in the “Select words to analysis” menu. “Stopwords” refer to numbers, symbols, be verbs, conjunctions, and adjectives that are not required for text data analysis (Zeng et al., 2023); these words were excluded from the text mining analysis. KH Coder provides a default stopword list file. In text mining analysis of research trends, research-related words such as “research,” “analysis,” “compare,” “evaluate,” and “properties” are usually considered stopwords (Park et al., 2023). Therefore, in this study, these words were added to the stopword list file.
Further, wood-science-related compound words such as “mechanical properties,” “elastic modulus,” and “dimensional stability” were extracted from wood science textbooks and added to the compound words file (Hoadley, 2000; Kollmann et al., 2012).
Word frequency analysis is a technique used to quantify the frequency of occurrence of words in documents. In such analyses, concepts including term frequency (TF), document frequency (DF), inverse document frequency (IDF), and term frequency-inverse document frequency (TF-IDF) are used.
TF is the number of times a given term appears in a document. The higher this value, the more critical the given term is in the document. DF is the number of documents in which a given term appears. IDF is the reciprocal of DF. TF-IDF is a weight obtained for each word in a document by using the word frequency and IDF. A word with a high TF-IDF value is likely to contain a key message in the document (Vijayarani et al., 2015). TF-IDF is widely used for extracting keywords from scientific papers (Toosi et al., 2022). In this study, it was used for word frequency analysis. The KH Coder only extracts TF and DF values. Therefore, TF-IDF was manually calculated using Equation (1) (Kim and Gil, 2019). For convenience, TF-IDF analysis is shown only for the top 50.
where N is the total number of documents (785 for JKWST, 812 for JWS).
Furthermore, a co-occurrence network analysis was conducted to evaluate the frequency of cooccurrence between specific words or objects within a given context. In a co-occurrence network, advanced natural language processing and text mining techniques are used to identify the most significant noun phrases in a document. These techniques create a visual representation of the interconnections between terms by forming a two-dimensional map. In this map, terms that frequently co-occur in the dataset are arranged close to each other, whereas terms that rarely co-occur are placed further apart (Mohammadi and Karami, 2022; Sakuma et al., 2021). The relationship between words is expressed in the form of a network connection. Through this, the main concept in the text can be identified, and the relationship between the concepts can be inferred (Yu and Rha, 2021). For the co-occurrence network for word analysis, the minimum TF value was set to 50 and minimum DF value was set to 10, and a network was created by connecting 100 pairs of the most strongly co-occurring words with a line.
3. RESULTS and DISCUSSION
Fig. 1 shows the TF-IDF analysis results from the abstracts obtained from JKWST. The most frequent word in the JKWST abstracts was “Pine,” and its TF-IDF value was 338.6. It was followed by “Korean,” “Dry,” “MDF,” and “CLT.”
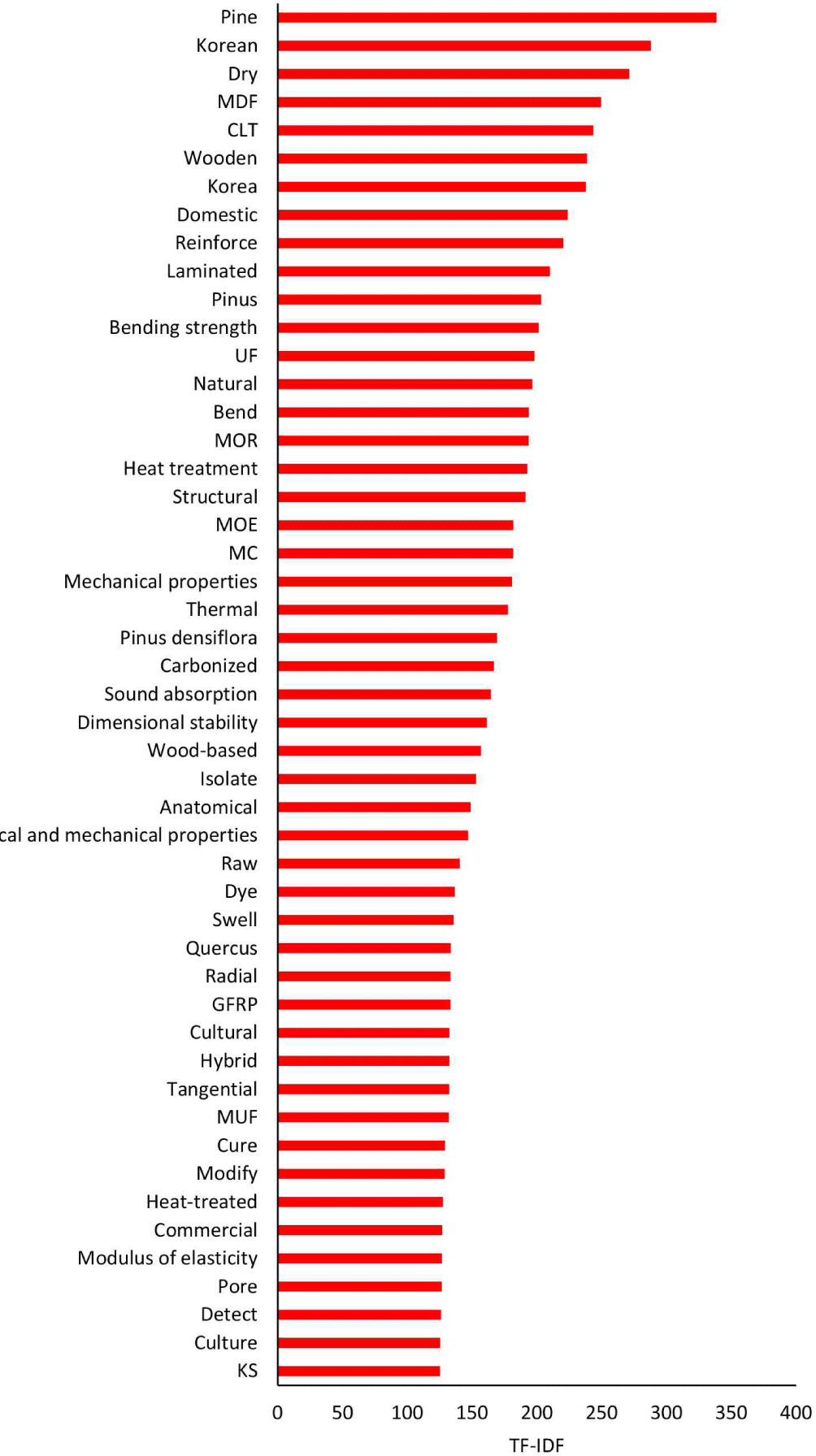
Along with “Pine,” “Pinus” (TF-IDF value: 203.3) and “Pinus densiflora” (169.3) were also ranked in the top 50. The most studied wood species in Korea was found to be pine (Pinus densiflora), followed by Quercus (133.6).
According to the 2020 Korea Forest Resources Report published by the Korea Forest Service (KFS, 2020), the area of pine forests in Korea is 1,272,000 ha; this is approximately 20% of the total forest area of 6,298,000 ha. Therefore, research on the use of domestic pine wood is needed urgently (Kim et al., 2008).
The words “Korean” (288.0), “Korea” (237.8), and “Domestic” (223.7) suggest that many studies were conducted on domestic wood species and wood industry. Words such as “Bending strength” (201.3), “MOR” (193.7), “MOE” (181.7), and “Mechanical properties” (180.8) were also ranked in the top 50, indicating that a large number of studies was conducted on the physical and mechanical properties of wood.
Among engineering wood materials, “MDF” (249.6) and “CLT” (243.4) were ranked high, and “MUF” had a score of 132.09. Unusually, glass fiber reinforced plastic (GFRP, 133.3) was also ranked in the top 50. This is a unique aspect of wood science research in Korea, and it reflects the fact that studies have actively investigated wood materials and GFRP composite laminates.
Among words related to wood modification, “Heat treatment” (192.6) had the highest frequency. Heat treatment involves the high-temperature heating of wood to induce changes in its chemical composition and thereby improve its dimensional stability, weather resistance, and permeability (Jang and Kang, 2022a; Kang et al., 2018; Kim, 2016).
“Sound absorption” (164.6) was also ranked in the top 50. This is because studies have investigated the sound absorption performance in terms of the porosity of wood and natural resources such as forest byproducts as sound absorption materials (Jang, 2022a; Jang et al., 2018; Jung et al., 2021; Kang et al., 2019).
Fig. 2 shows the TF-IDF analysis results from the abstracts obtained from JWS. In JWS, the word “Japanese” (192.3) was prominent, indicating the strong focus on studies related to the Japanese wood industry.
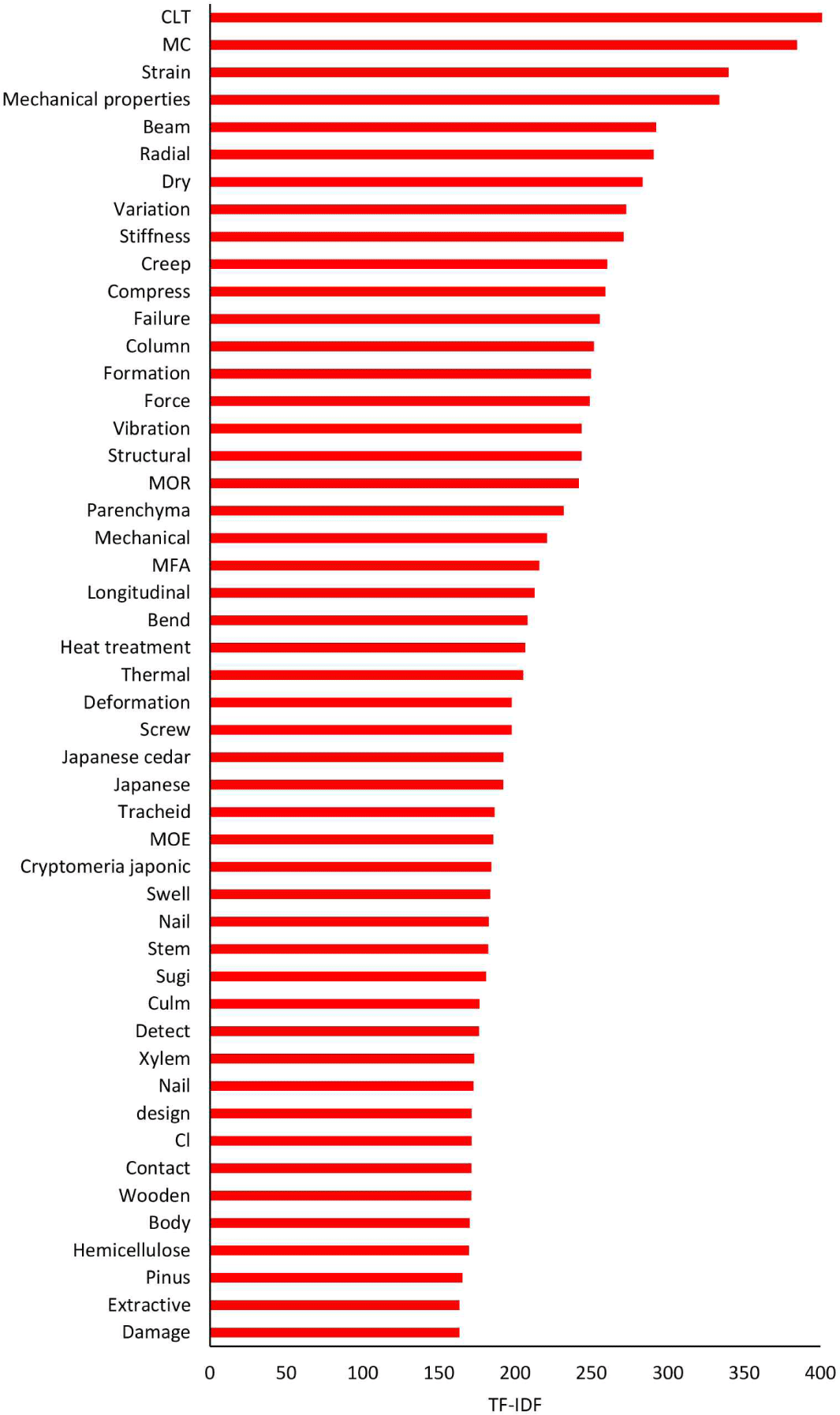
Furthermore, Japan-specific wood species showed high frequencies; for example, “Japanese cedar” (192.3), “Cryptomeria japonica” (184.4), and “Sugi” (181.02). “Pinus” was the next most commonly found keyword related to tree species in Japan. Accordingly, research on coniferous trees, especially Sugi and pine, was considered active in Japan.
Sugi (Cryptomeria japonica) is one of Japan’s most widely planted trees and has been cultivated in many parts of Japan for the past 400 years. Sugi plantations cover approximately 45,000 km2, accounting for 44% of Japan’s plantation forests (Kanasashi et al., 2015).
Terms such as “Strain” (339.9), “Mechanical properties” (333.8), “MOR” (241.7), and “MOE” (185.7) were present. Similar to Korea, a large number of studies focused on the physical and mechanical properties of wood.
As in Korea, wood materials such as “CLT” (459.1) were subjects of interest in Japanese wood research. Despite wood’s versatile application potential, these findings are likely influenced by the high frequency of CLT usage as a building material. “Heat treatment” was a common keyword related to wood modification in Japan as well.
In particular, wood anatomy terms such as “Parenchyma” (231.9), “MFA” (215.9), “Tracheid” (186.5), “Stem” (182.3), and “Xylem” (173.1) were ranked in the top 50. This suggests that wood anatomy research was likely conducted more actively in Japanese than in Korea.
Fig. 3 shows the results of co-occurrence network analysis conducted on JKWST. The analysis revealed that CLT is closely associated with terms like “structure,” “laminated,” and “reinforce.” This indicates a strong research focus on the adhesion and lamination of CLT (green group). The words “Pine,” “Korea,” “Korean,” and “Domestic” were part of the red group. Words related to physical properties, such as “MOR,” “MOE,” “Bending strength,” and “Dimensional stability,” were part of the blue group. They were organically related to the CLT group (green) and pine group (red). Furthermore, MDF is linked to terms such as “Carbonized,” “UF,” “Wood-based,” and “Sound absorption” (purple group).
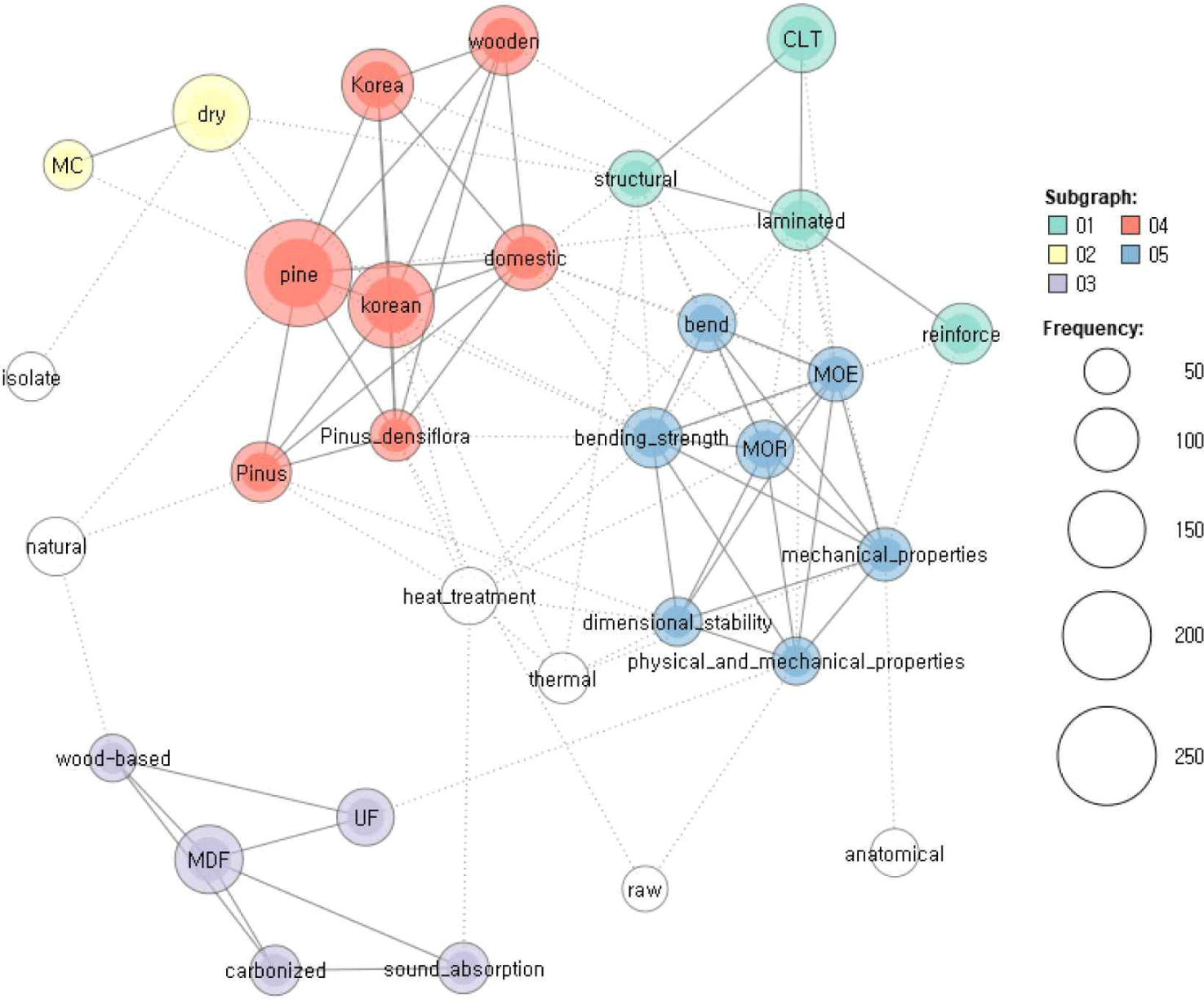
Fig. 4 shows the co-occurrence network analysis results in JWS. Similar to JKWST, JWS had five keyword groups. The keywords “Japanese cedar,” “Sugi,” and “Cryptomeria japonica” belonged to the blue group. This observation, similar to that in Korea, indicates the extensive research conducted in Japan on the use of native wood. The red group reveals a relationship between “mechanical properties” and terms representing physical properties, such as “MOR,” “MOE,” and “Bend.” This group has organic relationships with the green group containing words related to the structural properties of wood, such as “Failure,” “Stiffness,” “Force,” and “Deformation.” Further, these groups have been related to “CLT” The purple group consists of words like “Radial,” “Longitudinal,” “Parenchyma,” and “Stem.” This differentiation from JKWST suggests that studies on wood anatomy were published in JWS.
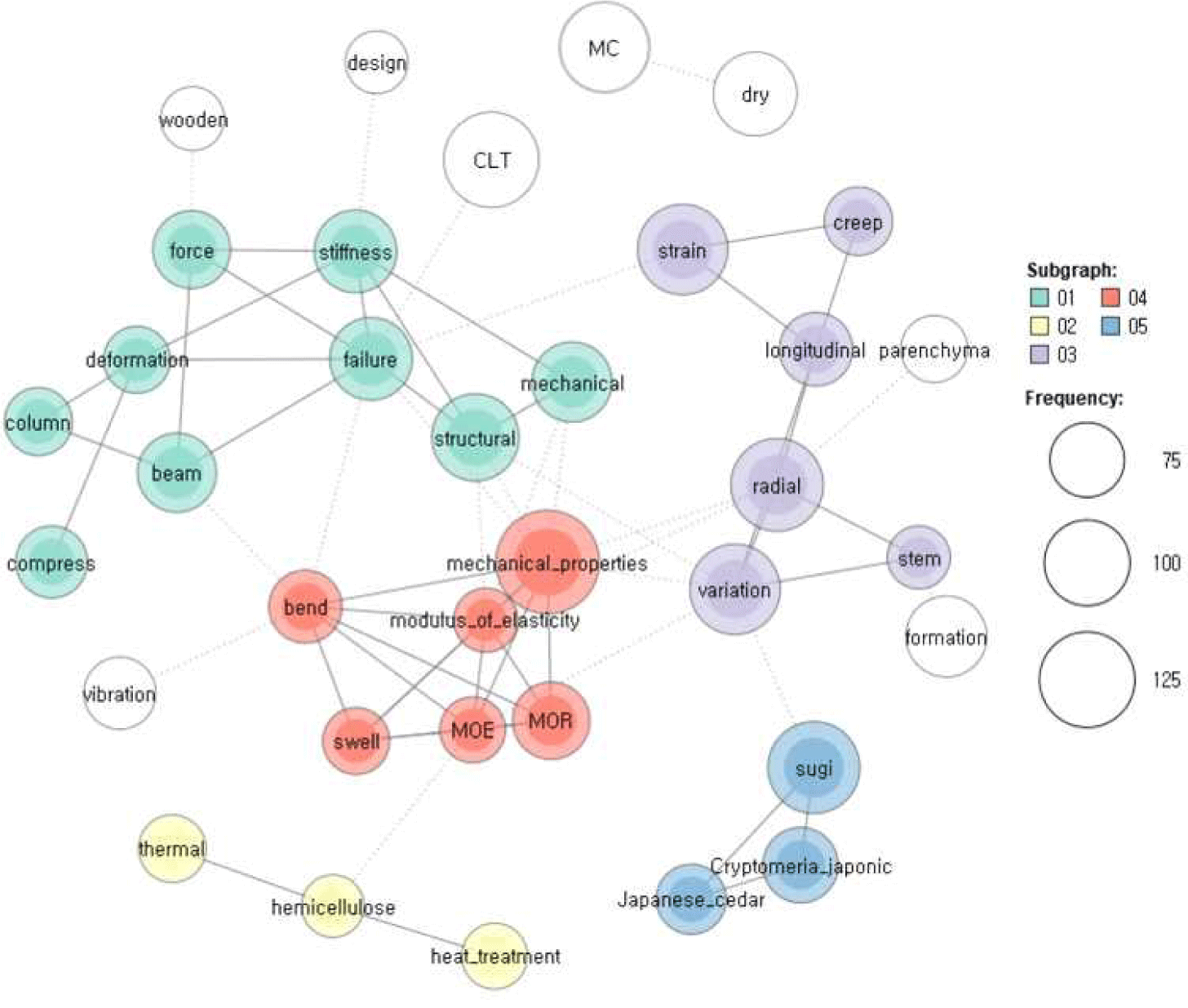
To the best of my knowledge, this study represents the first application of text mining analysis to wood science research papers. A limitation of this study is that the analysis only included wood science journals from Korea and Japan. In the future, text mining techniques for wood science can be expanded in numerous ways. For instance, they can be used to examine research trends in wood engineering across different countries or to track changes in wood science research topics over time. These endeavors are expected to open up opportunities for interdisciplinary collaborations within wood science and to pave the way for new research directions in this field.
4. CONCLUSIONS
In this study, text mining techniques were used to perform word frequency analyses including TF-IDF and co-occurrence network analysis by using abstracts published in JKWST (Korea) and JWS (Japan) between 2012 and 2022. The journals from both countries revealed a high frequency of keywords related to tree species native to the country and the country-based timber industry. Wood science studies in Korea and Japan were predominantly focused on the mechanical and physical properties of wood. CLT was a common research keyword in engineering wood-based materials in Korea and Japan. However, in the Korean study, the keywords “MDF,” “MUF,” and “GFRP” were also ranked in the top 50. Keywords related to wood anatomy appeared at a higher frequency in Japanese studies than in Korean ones. The analysis of co-occurrence networks revealed a strong organic relationship between words describing the physical and structural attributes of wood and the concept of wood materials.